By
Cortechs.ai
2 mins
By George Mastorakos, MD, MS
In the advent of 2023, NeuroQuant (NQ) 4.0 offers new features and continued segmentation / volumetric improvements to clinical workstations everywhere. Significant improvements to our Dynamic Atlas™️, enhanced gray matter segmentation, and the option of a modifiable contour object output are just a few highlights of NQ 4.0.
A major algorithmic change at the heart of NQ 4.0 is the combination of pre-existing, standard machine learning (ML) techniques with state-of-the-art deep learning (DL) medical image segmentation. As a brief note of comparison, ML and DL are two branches of artificial intelligence (AI) that are often used interchangeably, but there are key differences between them. ML is a method of teaching computers to learn from data, without being explicitly programmed. ML involves the use of algorithms that can analyze and interpret data to identify patterns and make predictions. DL, on the other hand, is a subfield of ML that involves the use of artificial neural networks, which somewhat mimic the structure and function of the human brain, to analyze and interpret complex data. Specifically, for image segmentation tasks such as brain structure segmentation, a special type of neural network is used: Convolutional Neural Networks (CNNs), which, you may have guessed, are convoluted! These CNNs are extremely powerful for identifying complex patterns in imaging data, thus making them a perfect fit for the task of accurate brain structure segmentation.
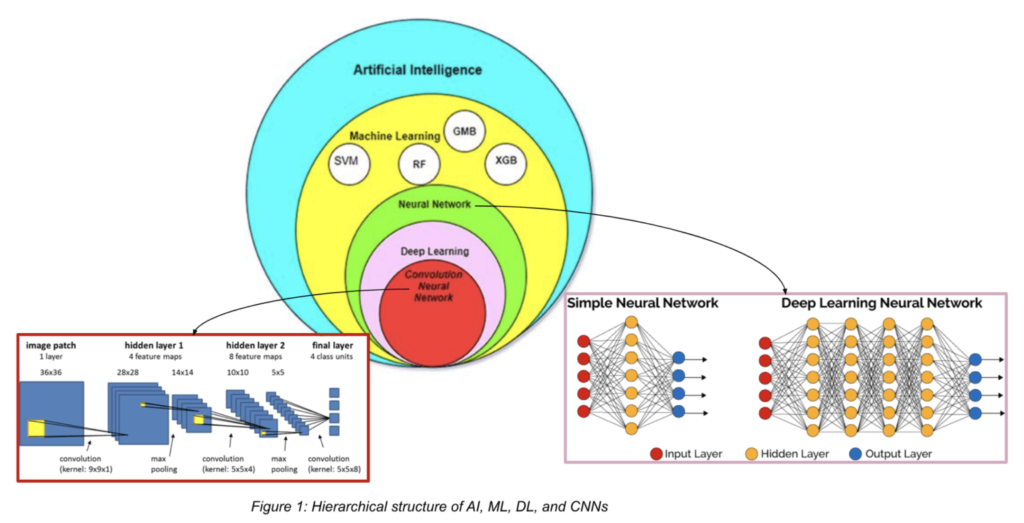
Previous versions of NQ relied solely on an ML approach and Dynamic Atlas™️ information to provide accurate brain structure segmentation. Typical challenging locations for segmentation included those near meninges, cortical structures, as well as distal cerebellar borders.
With NQ 4.0, a deep-learning based segmentation model was trained and layered atop previously used algorithms to create a hybrid, more robust model that segments 58 different brain structures. Notably, NQ 4.0 yields enhanced gray matter segmentation, as well as crisper segmentation boundaries at the meninges, cerebellopontine angle, and tentorium cerebelli. The power of DL can also be found in our other FDA-cleared product, OnQ Neuro, which segments brain tumors by subregion.
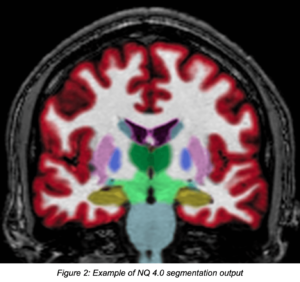
With the addition of this new and improved ML/DL combination algorithm approach, users can be assured that previous functionality of NQ remains available: few-minutes delivery of results straight to PACS, high-accuracy segmentation DICOM overlays, and customizable reports. Additionally, NQ 4.0 users can opt for the app to output enhanced segmentations as modifiable contour DICOM-compatible objects (Radiotherapy structure, or RT-Struct), which can be manipulated directly in PACS workstations.
Similar to the improvements outlined above for NQ, NeuroQuant MS (NQMS, formerly LesionQuant) lesion segmentation is now performed using a combination of standard machine learning and deep learning techniques.
NQ 4.0 brings a significant upgrade to the world of medical image analysis by combining the best of both ML and DL. The addition of DL algorithms has improved the accuracy and robustness of the overall brain structure segmentation model, without compromising key product offerings of the NQ system. We hope that these improvements in NQ and NQMS lesion segmentation continue to have a positive impact on clinical workstations and help medical professionals make more informed decisions in patient care.
Share